How SERVIR Uses AI to Turn Earth Science into Climate Action
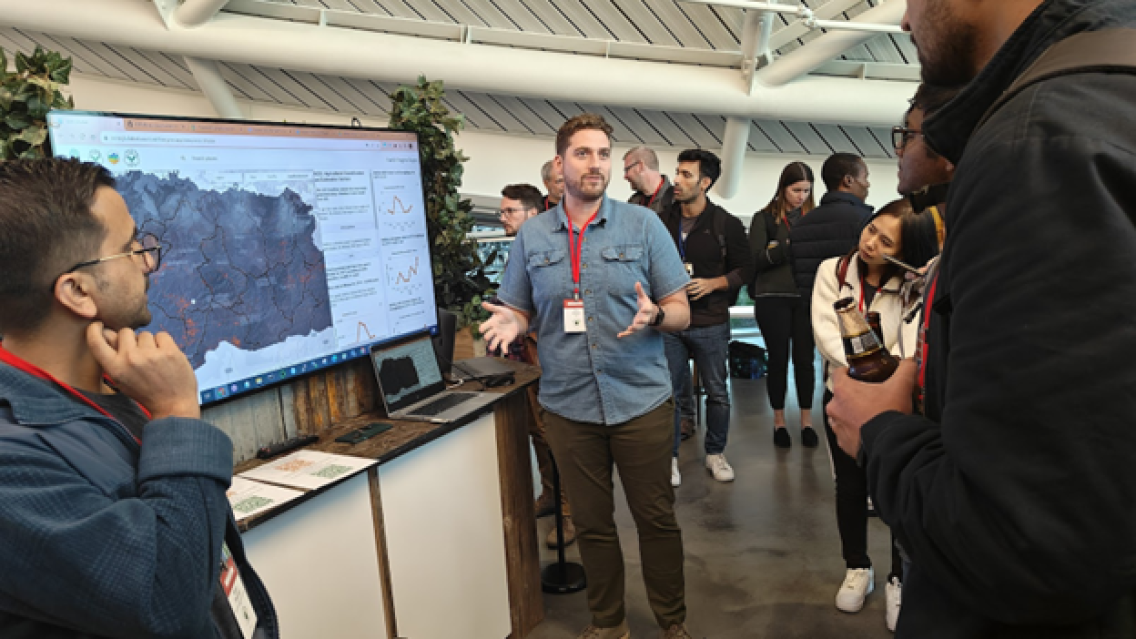
To study even a small piece of our planet, scientists often need to scour massive amounts of data. With artificial intelligence (AI), computers can assist humans in the complex and tedious task of interpreting Earth data–such as detecting deforestation or understanding climate patterns. AI is not a replacement for human expertise, but careful use helps SERVIR and its collaborators get more out of Earth data.
AI was a key theme at Google’s Geo for Good (G4G) Summit 2023 in Mountain View, California. G4G is an annual meeting of scientific and humanitarian organizations using geospatial technology and AI to support sustainability and climate resilience. G4G is an important opportunity for SERVIR to both share its achievements and collaborate on using Earth data to address real-world environmental challenges. This year, G4G was also a venue for data scientists from across SERVIR’s global network to share lessons learned with one another–especially when it comes to making the most of AI.
How Do We Use Artificial Intelligence in Earth Observation?
AI is designed to assist in tasks that are highly complex or time-consuming for people.
For example, a single Landsat 9 satellite image has over a million pixels. Each pixel has about a dozen “spectral” measurements – including different colors of visible light and thermal infrared–to help scientists identify different landscapes. To see how landscapes change over time, scientists often “stack” images from different dates–meaning every pixel can be compared across space and time. If a scientist compares Landsat 9 images of one location at ten different times, that’s ten sets of over a million pixels where each pixel has a dozen measurements. Even a fairly simple study may involve looking for subtle patterns in millions or billions of numbers. This is where AI becomes essential.
Without AI, computers can only detect patterns that humans specifically programmed them to find. With AI, programs can be designed to search for patterns more broadly. In massive amounts of data, AI can highlight a pattern even if the user didn’t program it to recognize that pattern specifically.
This basic form of AI is called machine learning and has been foundational to users of satellite imagery for decades.
For example, SERVIR’s Hydrologic Remote Sensing Analysis for Floods tool (HYDRAFloods) uses machine learning to map floods in near real-time. Without machine learning, a human would need to program to detect specific characteristics known about flooded pixels– "find pixels that look like water that didn’t look like water last week.” However, those instructions might miss muddy water, or flooding covered by trees. With machine learning, HYDRAFloods processes large amounts of satellite data to recognize other traits a human observer might overlook– "these pixels look different in infrared than they did last week.” This helps HYDRAFloods identify flooded pixels more reliably.
Example: Machine Learning to Detect Mining
At G4G, SERVIR highlighted several other examples of its AI use, including small-scale mining detection.
Surface mines cause deforestation and water pollution, and they often use child labor. However, they are hard to catch–they are constructed and abandoned very quickly in remote areas that are hard to patrol on the ground.
SERVIR Amazonia’s Radar Mining Monitoring tool (RAMI) uses data from radar satellites to “feel” the Earth’s surface and detect spots where mining activity has cleared the forest. Radar can sense deforested spots even through clouds. RAMI can identify the characteristics of a surface mine in radar data to spot mining activity. Users can verify what RAMI identifies and give it further feedback to help it improve.
RAMI builds on lessons learned from a service SERVIR West Africa designed with environmental agencies to detect similar mines, locally known as galamsey. SERVIR’s galamsey monitoring service in Ghana uses machine learning to help distinguish mines from other land clearing. It is also able to recognize abandoned mines to locate land restoration projects.
During G4G, SERVIR’s developers from South America and West Africa met in person and shared lessons learned from their approaches on tackling similar issues in both regions. Like the software itself, projects using AI succeed by incorporating feedback and learning in order to refine the outputs and their accuracy.
Making AI More Accessible
While AI has real ethical and practical concerns, it can be used carefully and responsibly to benefit Earth and climate action. By transparently documenting methods and uses, SERVIR can provide AI-based services that boost climate resilience, improve agriculture, and address air quality.
SERVIR collaborates with other NASA and USAID programs to create new, responsible uses for AI in Earth science. During G4G, SERVIR presented a general-purpose AI “Foundational Model” with the NASA IMPACT program. The model is designed to support many kinds of applications by new users. SERVIR strives to not only make wise use of AI itself but also to strengthen our partners’ capacity to use it, too.
Watch the SERVIR & NASA IMPACT session (on YouTube): Empowering Decision-Making with Google Tech + AI to Address Environmental Challenges.
-
Monitoring of Artisanal Mining (Galamsey) in Ghana
The Monitoring of Artisanal Mining (Galamsey) in Ghana Service is a monitoring platform with information on the location of illegal mining sites across Ghana and their associated land degradation.
-
Monitoring of Gold Mining in the Peruvian Amazon
The Monitoring of Gold Mining in the Peruvian Amazon service produces near real-time information on deforestation and mining activity in the southern Peruvian Amazon.